Structured Counterfactual Inference
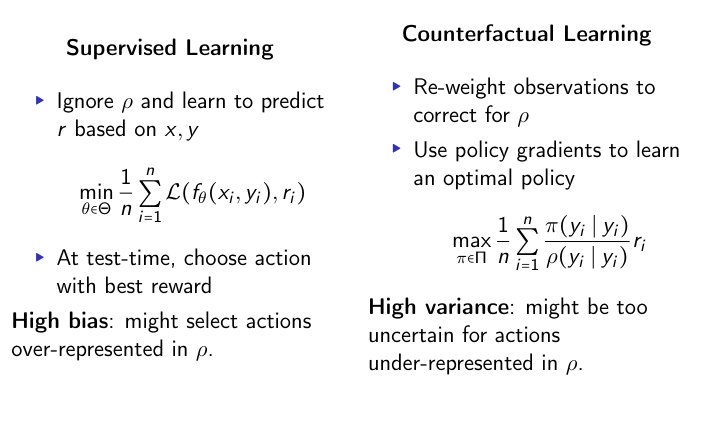
In batch learning from bandit feedback, we observe a context (e.g., some covariates describing a patient or a customer), choose an action (e.g., a treatment or a recommendation) and observe an outcome (e.g., treatment effect or a proxy for user experience). However, we may only observe the effects of a logging policy, that we hope to improve over. Dealing with biases and variance for offline policy learning is a challenging setting.
We study two specific scenarios of policy optimization in which additional structure (e.g., monotonicity or reward sparsity) can be exploited to provide better bias-variance trade-offs.
Publications
Learning from eXtreme bandit feedback
We study the problem of batch learning from bandit feedback in the setting of extremely large action spaces. Learning from extreme …
Cost-effective incentive allocation via structured counterfactual inference
We address a practical problem ubiquitous in modern industry, in which a mediator tries to learn a policy for allocating strategic …